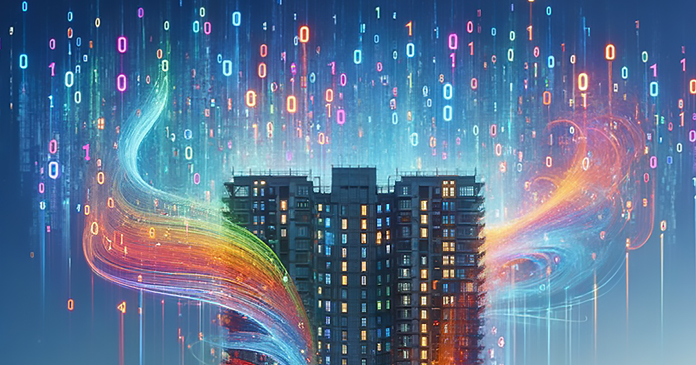
“In bear markets, stocks return to their rightful owners,” said J.P. Morgan. What Morgan was getting at is that generating strong returns during a bull market is essentially investing in “easy mode.”
And while a strong economy does not always guarantee good results, turbulent economic conditions often lay bare the difference between having a truly winning investment strategy and being a lucky hanger-on.
While the original quote specifies the stock market, the basic principle is clearly just as applicable to the real estate market. Just last year, property sale prices, as well as rental prices, were on a tear—rising at their fastest rate in decades in just about every American city.
Today’s picture is remarkably different, with all indications pointing to an oversupply of multifamily units and single-family homes. The result? Rental units are becoming harder to fill, and when leases do get signed, concessions are higher, and rents are lower. It is a perfect storm for property owners.
Meanwhile, general economic conditions—especially lingering inflation, despite central bank attempts to put the brakes on it—are in flux and unpredictable. Whereas a rental property that yields 4 percent would have been a solid investment last year, it’s hard to recommend that same investment today when mortgage rates have surged to 23-year highs.
Those who grew up in a robust real estate investment market that seemingly had no ceiling, may feel that the script has been flipped on them. Across the board, the realization is dawning that high-quality models—always important, to be sure—are indispensable amidst this uncertainty. Automated valuation models (AVMs) are already deeply integrated into the buy-and-sell real estate market, but when it comes to rental market investments, these computational tools do not reliably translate, leaving a major gap in the market.
What are needed are AVMs that reflect a more varied and subtle array of factors than buy-and-sell AVMs can accommodate.
A brief history of AVMs
AVMs play a critical role in today’s buy-and-sell residential real estate market. They work by taking a set of property characteristics and local market conditions from different databases and feeding them into computer-tabulated mathematical models to arrive at an estimated value for a given property.
The type of information fed into these models may include the property address and owner information, prior sales history, size of build property, lot size, construction year, number of bedrooms and bathrooms, data from locally available records such as deed transfers, recent sales of nearby comparable properties, and locally pertinent trends in pricing.
While AVMs have been in use since the 1990s, today’s AVMs have their origin in the late 2000s financial crisis, when overvalued home prices—and loans—led to broad value distortions in the property market. AVMs have quickly advanced in speed and accuracy in the years since the crisis, and today they offer buy-and-sell investors a powerful check on human bias and error in assessing property values.
The rental market, meanwhile, has lagged when it comes to the use of AVMs. One reason AVMs are used more frequently in buy-and-sell real estate than in the rental market is the lack of high-quality, readily available data. Sale prices of homes are a matter of public record and are registered as homes change hands, as are many other data points that feed AVMs. This gives AVMs pertinent and accurate information to make a reliable prediction.
Additionally, home prices have historically been less volatile than rentals, which are a more transient market. This is partially the result of renters’ motives: Some families may be committed to renting long-term, whereas others see renting as a temporary steppingstone to purchasing a home.
Ultimately, rental market investments are determined by far more complex forces than buy-and-sell. For this reason, rental investors and lenders have often relied on hedonic methods, whereby regression is used to assess property value by slicing the asset into constituent parts and features then assigning a value and weight to each respective characteristic. Standardized models as well as macro, micro, and spatial factors are also used.
Capturing this type of data in an AVM requires a vast and comprehensive database. This database must be robustly maintained and updated in real time. To be accurate on a national level, a database that sufficiently feeds an AVM to determine rental prices must encompass data on tens of millions of built properties in diverse markets—from big cities to small towns—and more than 100 million land parcels.
The machine learning fix
Machine learning (ML) is bringing speed, accuracy, and robustness to rental AVM models where they have historically been lacking. Using machine learning, predictive models are being developed that can adapt and improve their functioning over time without requiring explicit human input.
These algorithms and statistical models tap into vast and varied pools of data and analyze that data for deep insight. The advantage of ML over traditional computation methods is already well recognized and ML is in play in almost every major sector—from healthcare to heavy industry.
In the real estate world, ML is used to narrow the margin of error and accelerate price estimates where quick and accurate results are necessary to move forward with transactions. While traditional appraisals are needed at some point down the line, having an accurate estimate upfront provides several benefits to lenders and equity investors.
Recent research has found that machine learning methods provide comparable accuracy to the hedonic pricing approach yet are more flexible when it comes to finding relationships between variables and their ease of implementation. Such models, by offering better information symmetry between prospective tenants and landlords, are promising new efficiency gains in the rental market.
With transactions at a near standstill due to today’s economic uncertainty, innovative AVMs offer a powerful tool to help single and multifamily rental investors better assess potential investments in single and multifamily rental units. These models owe their accuracy in large part to their ability to source and leverage massive amounts of pertinent data, including current and historic listing data, rent rolls collected from lenders, other AVM users, and client data.
This information includes current and past resident payment history, property and unit features and occupancies, applicant history, and neighborhood information culled from sources such as the U.S. Census Bureau, noise, pollution, and crime stats, as well as school district and geographic details.
Looking forward
Up until now, the power of AVMs has primarily benefited transactions in the real estate ecosystem through home and apartment values.
With machine learning-powered rental AVMs, investors can make better decisions about single and multifamily projects, where anticipating rental value is vital, while lenders looking for an objective understanding of the real estate market can apply yield metrics to compute asset valuation.
This is particularly important in the wake of the material drop in transaction volume in recent months. The conditions of the economy are impossible to predict, which is putting investments in the real estate world in flux. With highly accurate and thoroughly tested rental AVMs, it is possible to make informed decisions on whether to pursue a given project.
With a drop in rents across the U.S. for multifamily and single-family rentals, these tools are needed now more than ever. And as these AVMs gain access to more and more datastores, their accuracy will only continue to improve.